LATEST RESOURCES
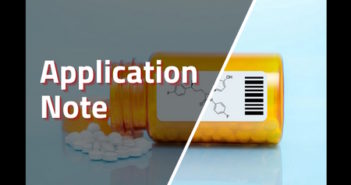
In this application note, Prof. Rafael Cela, showcases the selection of LC procedures using Mgears Chrom Best Method and highlights how automation streamlines the screening process and helps in analysis, reporting, and decision-making…
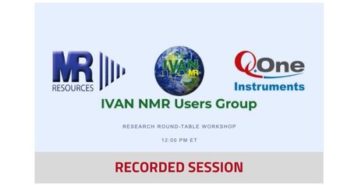
Watch the recorded session of our colleagues, Mark Dixon and Marcel Lachenmann from Mestrelab Research, speaking at the last IVAN Research Round-table Workshop held in February 2024!

In this insightful exchange, we are privileged to have John, a distinguished expert with over 40 years of experience in the scientific and informatics domains, particularly within the pharmaceutical industry.

The U.S. Pharmacopeia (USP) and Mestrelab Research, S.L. (Mestrelab), are pleased to announce they have entered into a collaboration to deliver USP-ID, through Mestrelab’s Mnova platform…

We are excited to introduce our Mnova Purification Suite, meticulously crafted to offer flexible, modular solutions that address the critical aspects of chromatographic purification…

Introduction Spectroscopic techniques, particularly NMR, frequently encounter the challenge of overlapping peaks. Thus, the need to enhance the resolution…

In the world of spectral data, the struggle to access and manage diverse datasets across various platforms is real. That’s why we’ve developed Mnova Hub…


Molecular Melodies: Unraveling the Hidden Harmonies of NMR Spectroscopy
Title: Molecular Melodies: Unraveling the Hidden Harmonies of NMR Spectroscopy Authors: Iria Pérez Varela, Gavin Shear, Carlos Cobas Date: 7…